"WANTED. DESIRED. ETERNAL. YOUTHFUL." FOREVERWANTEDCLUB THE FASHION & POP CULTURE MAGAZINE
Shadowbanning, Content Ranking, Reels & Growth: Adam Mosseri Reveals How The IG Algo Ranks Content
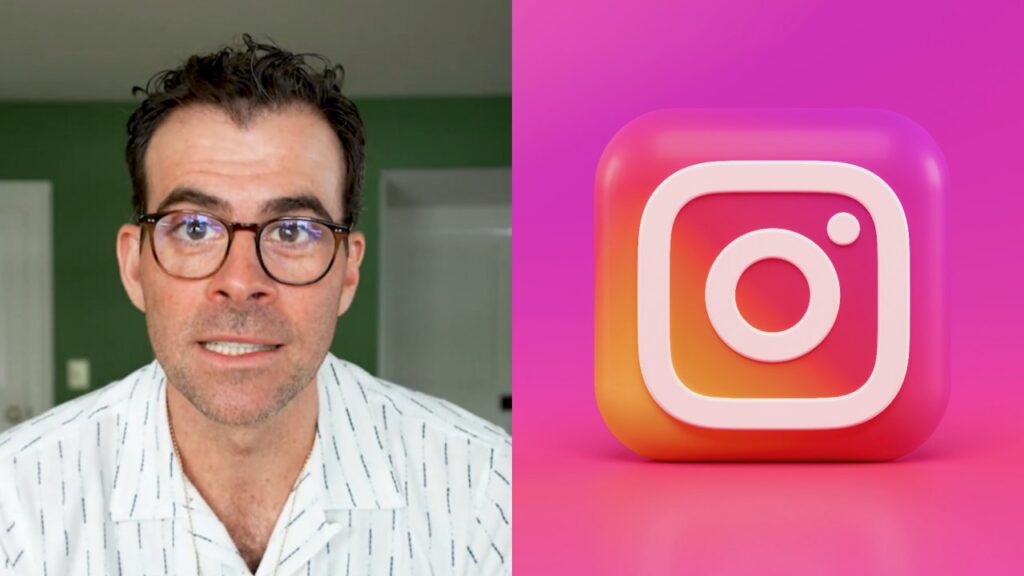
Instagram users have long been curious about how the platform’s algorithm determines the content they see and why certain posts appear more prominently than others. Now, Instagram is shedding some light on its recommendation system, providing insights into why specific posts are shown, although the explanation is partial. In a recent blog post, the company outlines how its ranking system operates across different sections of the app, including the main feed, Stories, the Explore page, and Reels.
Instagram utilizes a multitude of data points to predict user preferences and interactions, emphasizing that there isn’t a single all-encompassing algorithm behind recommendations. Unsurprisingly, a significant portion of the data used for content ranking comes directly from user activity. For instance, Stories are ranked based on factors such as a user’s frequency of viewing an account’s updates and engagement through actions like sending direct messages or liking Stories. Instagram also takes into consideration the user’s relationship with an account, including whether the account belongs to a family member or friend.
When it comes to Reels, recommendations are driven by similar user data. Instagram reveals that a user’s likes, saves, shares, and interactions shape the content they will be presented with next. The platform explicitly mentions factors like the likelihood of resharing a video, watching it until the end, liking it, or engaging with the audio page as key predictors. Visual and audio elements within the videos, as well as information about the account that shared them (such as follower count and engagement levels), also contribute to the ranking of Reels.
Platforms like Facebook and TikTok offer users various tools to control their content preferences. Facebook’s Meta has introduced “show more” and “show less” options, allowing users to respond to specific types of content. On TikTok, users can “dislike” videos and create lists of hashtags and words they prefer not to encounter in their feeds.
The effectiveness of these tools, however, remains questionable, with some studies suggesting that user settings aimed at fine-tuning recommendation systems have limited impact.